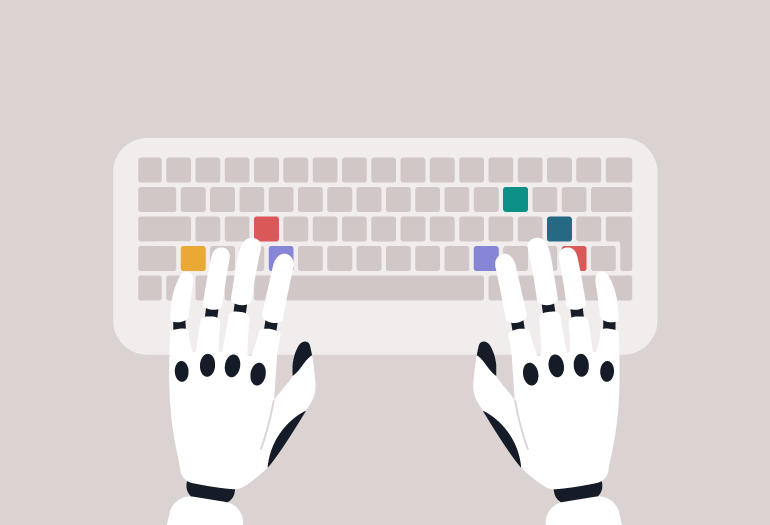

ChatGPT = Chat Generative Pre-training Transformer
ChatGPT is an AI chatbot developed by OpenAI that exploded into mainstream consciousness shortly after it was released in November 2022. It’s not the first chatbot of its kind, but thanks to its advanced capabilities and impressive human-like responses it’s certainly the most popular right now.
There are endless potential applications of this kind of technology, but at Profitero we’ve been investigating how you can use ChatGPT for free analysis of data collected on our platform.
Note: To write this guide we asked ChatGPT several specific questions, and in some instances the exact output is displayed below. Apart from screenshots, you can easily spot the ChatGPT output as the text is formatted in italics.
How is ChatGPT relevant for ecomm geeks?
To understand how we can begin to harness the power of ChatGPT for digital shelf analytics, we asked the expert itself:
Q: Explain what ChatGPT is as if you are talking to an audience of eCommerce analytics leaders.
A: Hello, eCommerce analytics leaders! I am ChatGPT, a large language model trained by OpenAI, based on the GPT-3.5 architecture.
In simpler terms, I am an AI language model designed to understand and generate human-like language. I have been trained on an enormous amount of data from the internet and various other sources, which has allowed me to develop a deep understanding of language patterns, grammar, and vocabulary.
As an eCommerce analytics leader, you may be interested in knowing that I can help you analyze and understand your customers' behavior through language processing. This includes analyzing customer reviews, social media posts, and other forms of customer feedback to gain insights into your customers' preferences, needs, and pain points. With this information, you can improve your products and services to better meet the needs of your customers.
Furthermore, I can help you improve your customer support by providing you with a powerful chatbot that can interact with customers in natural language, answer their questions, and provide personalized recommendations. This can help you enhance the customer experience, reduce support costs, and increase customer satisfaction.
Overall, as an eCommerce analytics leader, you can leverage my capabilities to gain valuable insights into customer behavior and enhance the customer experience through advanced natural language processing.
Keep reading for 4 step-by-step guides on how to use ChatGPT in partnership with Profitero data for a quick (and reasonably accurate) analysis of consumer product reviews.
Disclaimer: Before we dive in, this article is based on our experimentation with the free version of ChatGPT. The enterprise version, particularly GPT4, has much more advanced capabilities, including expanded word limits and even image analysis.
It’s well documented that the free version of ChatGPT isn’t perfect and will have some inherent biases.
When asked on its limitations for consumer review analysis, ChatGPT says:
While ChatGPT can be a powerful tool for analyzing consumer reviews, there are some limitations to keep in mind. Here are a few:
-
Context: ChatGPT is trained on a vast amount of data, but it may not always understand the context in which a review is written. For example, it may not be able to distinguish between positive and negative reviews if the context is unclear.
-
Bias: The data that ChatGPT is trained on may be biased towards certain demographics or types of products, which can impact the accuracy of its analysis.
-
Incomplete Data: ChatGPT may not have access to all the necessary data to analyze consumer reviews, especially if the data is not available in a structured format.
-
Subjectivity: Consumer reviews can be highly subjective, and ChatGPT may not always be able to accurately interpret the meaning behind a review.
-
Limited Vocabulary: While ChatGPT has a large vocabulary, it may not always be able to understand the specialized language and terminology used in some reviews, particularly in niche industries.
It's important to keep these limitations in mind when using ChatGPT for analyzing consumer reviews. It's always a good idea to combine the insights generated by ChatGPT with other sources of data and human analysis to get a complete picture of customer feedback.
#1 — Review text sentiment analysis
One of the easiest ways to harness the power of ChatGPT is to derive sentiment analysis from your consumer product reviews. This is as simple as framing your request in the right way and pasting in some data. It doesn’t require specialist programs or coding knowledge — all you need is access to ChatGPT and a spreadsheet containing the review text you want to analyze.
Example input:
Example output:
Step-by-step guide:
-
Export Reviews data from the Profitero app.
-
Type into the chat: “please* provide one word text sentiment analysis for these X pieces of text in a table format that I can copy and paste into a spreadsheet:” (where X is the number of rows you are processing). Don’t press Enter yet.
-
Paste the Review Text column values into the chat, then press Enter.
-
The bot should give a one-word sentiment analysis for each piece.
-
If the format isn’t ideal, you can click “re-generate response”.
-
Copy and paste the table back into Excel.
*The “please” is optional, but we think it’s a safe bet to treat potential future overlords with some politeness.
Profitero pro tips:
-
There is a character limit to ChatGPT inputs & outputs: the free version is the most restricted, but this is less of an issue with the Enterprise version. In our tests we have found that free ChatGPT will usually analyze 10-25 reviews in each request. Bear that in mind before you download your data sample.
-
The ChatGPT sentiment analysis can support a number of different outputs, including:
-
-
Polarity-based analysis: This technique involves classifying the sentiment of a text as either positive, negative or neutral. It involves using a lexicon or a dictionary that contains a list of words with their corresponding polarity scores. (used in the example above)
-
Aspect-based analysis: This technique involves identifying the various aspects of a product or service that consumers mention in their reviews, and then analyzing the sentiment associated with each aspect.
-
Emotion-based analysis: This technique involves identifying the emotions expressed in the consumer review text, such as happiness, sadness, anger, fear, and disgust.
-
Intent-based analysis: This technique involves identifying the underlying intent of the consumer review, such as a complaint or a suggestion, and then analyzing the sentiment associated with that intent.
-
3. To access different types of sentiment analysis, change the command above from “one-word text sentiment analysis” to either:
-
"aspect-based analysis"
-
"emotion-based analysis"
-
"intent-based analysis"
#2 — Review text topic categorization
As well as sentiment analysis, ChatGPT can also be used for text topic analysis. There are advanced capabilities that we will break down below, but if you know the rough topics you expect to see in your reviews, you can use ChatGPT to quickly and easily sort them. As with sentiment analysis, this doesn’t require specialist software or knowledge, just access to ChatGPT and a spreadsheet containing the review text you want to analyze.
Example input:
Example output:
Step-by-step guide:
-
Export reviews data from the Profitero app.
-
Type into the chat: “please classify these X reviews into N categories: Category1, Category2, Category3,....CategoryN. Reviews:” (where X is the number of rows you are processing, N is the number of categories you want to use and Category1…CategoryN are the categories). Don’t press Enter yet.
-
Paste the Review Text column values into the chat, then press Enter.
-
The bot will return a table with your reviews in one column and one of your predetermined categories in the other column.
-
If the format isn’t ideal, you can click “re-generate response”.
-
Copy and paste the output back into Excel.
Profitero pro tips:
-
As before, this analysis will be subject to character limits, so you can’t use it to analyze a vast amount of data in one go. You may need to split your data into several chunks.
-
The categorization is only as good as the topics you give it, and it’s a good idea to include an “other” category so the bot has somewhere to put reviews that don’t easily fit anywhere else.
-
This analysis was noticeably slower than the one-word sentiment analysis, but still quicker and more convenient than manual analysis.
#3 — Advanced review text topic modeling (tokenization)
When it comes to more advanced kinds of text topic analysis, ChatGPT can help get you started, but this kind of work is best done by a qualified data analyst with experience working with Natural Language Processing techniques. Here is what we found when we asked ChatGPT the question:
Q: How can I use ChatGPT for topic modeling on consumer reviews?
A:
-
Preprocessing: Clean the text data by removing stop words, punctuations, and special characters. Convert all the text to lowercase and remove any irrelevant information like usernames, URLs, and numbers.
-
Tokenization: Tokenize the text by splitting the sentences into individual words or phrases. You can use the NLTK library or Spacy for this purpose.
-
Embedding: Use pre-trained language models like GPT-3 to embed the tokens. This step is essential to capture the semantic meaning of the words.
-
Topic Modelling: Apply topic modelling algorithms like Latent Dirichlet Allocation (LDA) or Non-negative Matrix Factorization (NMF) to the embedded tokens to identify the topics. These algorithms group the tokens based on their co-occurrence and assign weights to each topic.
-
Interpretation: Interpret the results by analyzing the top keywords and the weight of each topic. You can use visualization tools like pyLDAvis or Gensim to visualize the topics.
ChatGPT claims it can be used for steps 2 & 3. In our experience, it is reasonably simple to use ChatGPT for tokenization, but from this point onwards the outputs need to be interpreted and used by an experienced analyst — and ChatGPT would be much less efficient than using a coding language such as Python.
Example input:
Example output:
Step-by-step guide:
-
Type into the chat: “please tokenize the following X pieces of review text for topic modeling and output in a table format that I can copy and paste into a spreadsheet:” (where X is the number of rows you are processing). Don’t press enter yet.
-
Paste the Review Text column values into the chat, then press Enter.
-
The bot should provide a table with the Review Text in one column and Tokenized Text in the other.
-
If the format isn’t ideal, you can click “re-generate response”.
-
Copy and paste the table back into Excel.
Profitero pro tips:
1. If you need a handy Excel formula to help you with preprocessing your data, try:
=LOWER(TRIM(SUBSTITUTE(SUBSTITUTE(SUBSTITUTE(SUBSTITUTE(SUBSTITUTE(SUBSTITUTE(SUBSTITUTE(SUBSTITUTE(A1,"("," "),")"," "),"-"," "),":"," "),";"," "),"!"," "),","," "),"."," ").
Where A1 is the cell with your review text.
2. These more advanced processes are where the character limit and speed of ChatGPT prove most inconvenient. This was by far the slowest response time of all of our tests.
3. As the tokenized data still needs further processing outside of ChatGPT, this is an example where just because you CAN use ChatGPT, doesn’t mean you SHOULD.
#4 — Advanced review text topic modeling (Python code)
Rather than using ChatGPT to perform parts of the text topic modeling, a more valuable use of time is to get ChatGPT to write code in a language such as Python that will perform all of the steps involved. ChatGPT’s ability to write simple code can be a hugely beneficial time-saving device for data analysts. We tested Python code for our use case, but ChatGPT can also write code for:
-
JavaScript
-
C++
-
C#
-
Java
-
Ruby
-
PHP
-
Go
-
Swift
-
TypeScript
-
SQL
-
Shell
Here’s an example of how you can use ChatGPT to generate Python code to do the full text topic analysis.
How to structure your data:
Save your review text in a CSV file with a single column with the header “Review Text”.
Example input:
Example output:
We’ve tested this code and any analyst familiar with Python should be able to adapt it and run it with no problems.
Profitero pro tips:
-
ChatGPT is not a replacement for the data analysts on your team. The outputs are a good starting point, but it still takes a skilled data analyst to amend and execute the code.
-
The key to using ChatGPT to write code successfully is to keep your requests simple. The more complicated your request, the more likely you are to spend more time debugging than it would have taken an analyst to write the code from scratch.
So what? Now what?
Here are our closing thoughts on using the free version of ChatGPT for review text analysis:
The Good
-
Experimenting with ChatGPT was incredibly intuitive — it didn’t take much iteration between the first attempt at asking a question to receiving an output that was pretty close to the ideal.
-
The accuracy is pretty good! In the one-word sentiment analysis we tried, 90% of reviews that ChatGPT marked as Negative were 1- to 2-star examples & 90% of reviews that ChatGPT marked as Positive were 4- to 5-star examples. Neutral is a more of a gray area — only 41% of the reviews that ChatGPT marked as Neutral or Mixed were 3-star examples.
-
We tested examples from the U.S., U.K., Germany & France and found the accuracy was equally good across the U.S., U.K. & Germany, but French language analysis had reduced accuracy: only 78% of reviews marked as positive originally had a 4- or 5-star rating. When asked about language support, ChatGPT says:
ChatGPT can process text in many languages, including English, Spanish, French, German, Italian, Portuguese, Chinese, Japanese, Korean, Arabic, and many others. However, its level of proficiency and accuracy may vary depending on the language and the amount of training data available for that language.
The Challenges
-
In the free version we used, the character limitation is a huge blocker. In our tests, on average ChatGPT would analyze a text sample of around 2,300 characters — the minimum it processed at once was 1,024 and the maximum was 3,825, which is close to the reported maximum from other sources. If you’re serious about using ChatGPT for this kind of analysis, there is a paid Enterprise API option which may be helpful.
-
Sometimes the sentiment analysis output truncated the text in the original review — the good thing about the limited input is that it’s easy to verify by eye which sentiment matches each original review.
-
The free version was not reliably available, and we did run into occasions where ChatGPT was “at capacity”. This was most noticeable when the east coast U.S. started to wake up (8-9am ET), and we had fewer issues during EMEA morning periods (8-11am GMT).
-
ChatGPT can be unpredictable. When we translated the sentiment analysis request into French (when processing French language reviews), we did not receive the same 3-word scale that we got with the English language request. Instead, we got 19 different one-word summaries (based on a sample of 25 reviews). ChatGPT was also unpredictable in terms of how much text it would analyze at once.
-
It may be the market leader in terms of AI chatbot technology, but it’s not the market leader for text analysis or digital shelf intelligence. If you don’t have the time or expertise to play around with the output from ChatGPT, you are better off investing in Profitero’s advisory services, which can help you unlock the insight hidden within your digital shelf data and much more!
Learn more about Profitero's suite of advisory services here or contact one of our expert coaches directly by emailing strategicsolutions@profitero.com.